INNS Webinar Series Archive
Explore our archive of past INNS Webinars. This rich collection provides a valuable learning resource for students and professionals interested in neural networks and related research.
Dive into recordings of previous lectures from our bi-monthly Webinar Series. For information on upcoming live sessions and the overall series, please visit the INNS Webinar Series page.
2025
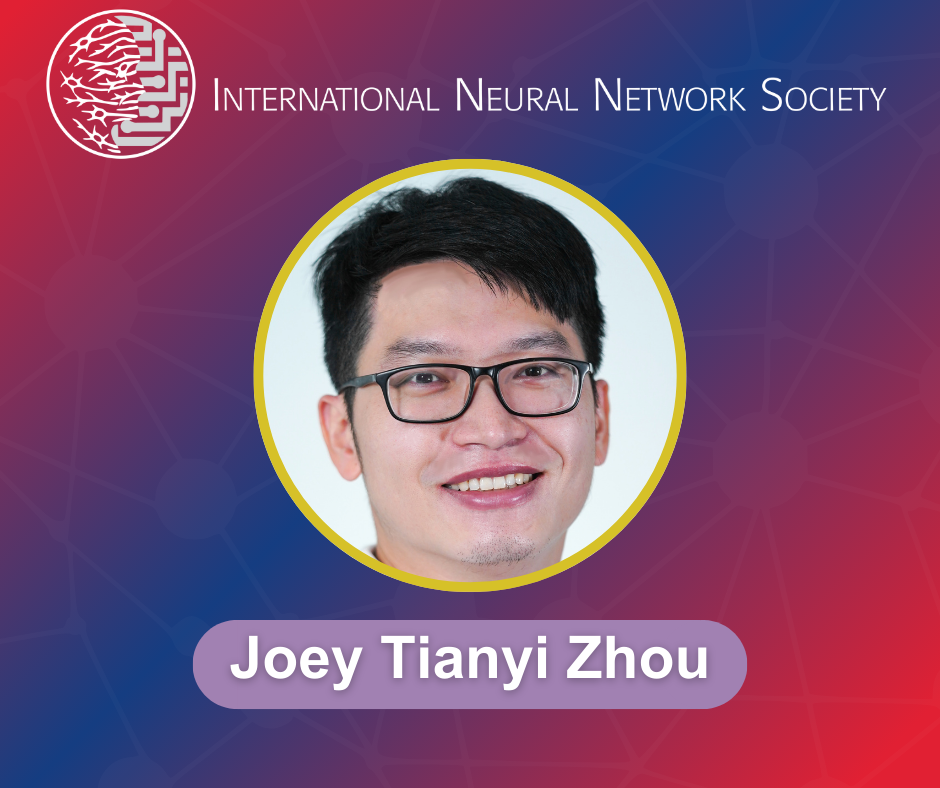
Dataset Distillation and Pruning: Streamlining Machine Learning Performance
Joey Tianyi Zhou
25 April 2025
Abstract: In the rapidly evolving field of machine learning, "Dataset Distillation and Pruning" has emerged as a key strategy for enhancing model efficiency. Dataset distillation involves extracting essential information from extensive datasets to create refined, smaller-scale data that maintains model robustness while reducing computational burden. It can be likened to distilling knowledge from vast amounts of data. On the other hand, dataset pruning is akin to pruning unnecessary branches from a tree. This technique involves removing redundant or minimally impactful data points, resulting in a more streamlined, faster, and resource-efficient machine learning model. By eliminating extraneous information, dataset pruning aids in constructing lean algorithms with outstanding performance and without unnecessary computational overhead. These two approaches collectively address the challenges posed by the abundance of data in the digital age. Dataset distillation and pruning complement each other in model compression research and further optimize the entire machine learning workflow's energy consumption, ultimately facilitating sustainable deployment of large-scale data and models on endpoints.
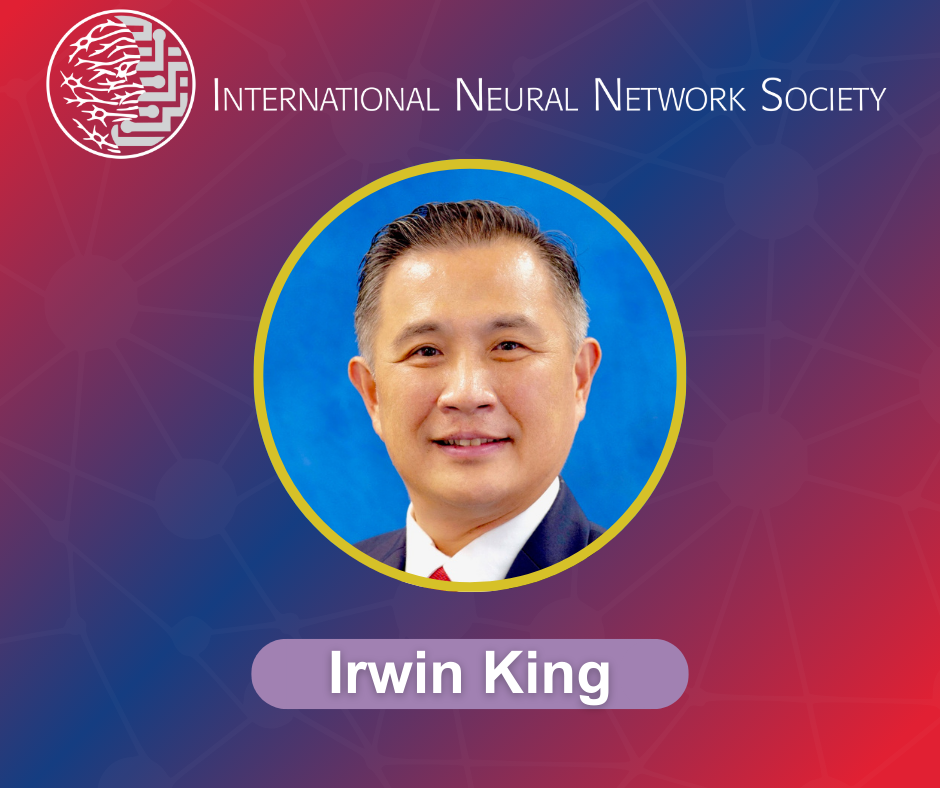
The Critical Role of AI in Learning Analytics and Assessment in the Future of Education
Irwin King
10 April 2025
Presentation Slides
Abstract: The increasing adoption of Artificial Intelligence (AI) in higher education presents both opportunities and challenges for institutions, teachers, and students. As AI-driven tools for personalized learning and alternative assessment approaches are poised to replace or transform traditional methods, this presentation delves into the transformative impact of AI on the future of education. We will explore current trends in learning and assessment, examining how AI technology is redefining these practices. This presentation aims to provide a comprehensive understanding of how AI is reshaping assessment practices and driving the future of educational success, catering to learners, educators, administrators, and policymakers.
2024
INNS Annual Lecture Nobelization of Neural Networks: Deep Roots and Insane Future of Neural Networks
Wlodzislaw Duch
17 December 2024
Presentation Slides
Abstract: The 2024 Nobel Prizes in Physics and Chemistry highlighted the pivotal role of neural networks in scientific advancement. John Hopfield’s foundational work is deeply rooted in statistical physics, tracing back to the one-dimensional Lenz-Ising model of ferromagnetism (1924). Subsequent development of the Ising model contributed to four Nobel Prizes: the two-dimensional model was solved by Lars Onsager (1968), models of spin glasses were developed by Philip Anderson (1977) and Giorgio Parisi (2021), and model dynamics was investigated by Roy Glauber (2005). This research led to the complex systems theory and the emergence of self-organizing associative memory systems, as explored by Steve Grossberg (1969), Shun-ichi Amari (1972), and others. John Hopfield connected these ideas to statistical physics (1982, 1984). Geoffrey Hinton pioneered the methods to learn internal representations of information in complex networks, contributing to the wide acceptance of the backpropagation algorithm (1986), Boltzmann machines (1985), and deep learning advancements (2015). This work has spurred remarkable progress in machine learning, including the advent of physics-informed machine learning (PIML) and recent ideas in the physical implementation of probabilistic machine learning. From these theoretical foundations, great advancements in artificial intelligence, exemplified by the success of Deep Mind’s AlphaGo program in winning with world champions, emerged. DeepMind is a company founded by Demis Hassabis, a computational neuroscientist who combined insights from systems neuroscience, machine learning, and computing hardware to "solve intelligence" and apply it to various complex challenges. Significant breakthroughs, such as the AlphaFold series of programs, effectively addressed a 50-year challenge in biophysics by predicting 3D protein structures with high accuracy from their 1D amino acid sequences. We now possess tools to analyze the behavior of complex systems that are computationally irreducible. The social implications of these developments are profound and unpredictable. Many new developments are introduced with unprecedented speed, leading to agents based on large multi-modal systems that are autonomous, can reason, have a long-term memory, are creative and understand human psychology. Recent developments indicate that such models have internalized substantial knowledge about the world and demonstrate various emergent behaviors. The evolution of agents based on such models, capable of self-reflection, points toward a form of digital intelligence that may deserve the status of digital beings. This year's Nobel Prizes should prompt us to reflect on the transformative wave of machine learning methods shaping our future.
AI for Finance: A Practitioner's Viewpoint on Using Neural Networks to Forecast Equity Returns
Ryan Samson
12 December 2024
Presentation Slides
Towards Lifelong Learning Intelligent Agents Capable of Focusing Attention and Taking Conscious Actions - Neural Propagation in the Framework of Cognidynamics
Marco Gori 7 November 2024
Abstract: The fields of Artificial Intelligence (AI) and Cognitive Science began intersecting significantly during the Eighties when the Connectionist wave strongly propelled studies on Artificial Neural Networks. The evolution of AI over the last few decades, focusing on deep learning and, more recently, generative AI, has produced spectacular results that were hardly predictable even by the pioneers of the discipline. However, when examining early studies on Connectionism, many aspirations remain unrealized, as most successful outcomes rely on the brute force of combining computational resources with large data collections. This stands in contrast to nature, where cognition emerges from environmental interactions and the processing of temporal information. In order to capture those natural processes and explore an alternative path to Machine Learning, in this talk I introduce the framework of Cognidynamics that describes cognitive systems whose environmental interactions are driven by the minimization of a functional over time. This functional, referred to as cognitive action, replaces the traditional statistical functional risk of Machine Learning in the temporal dimension. I employ the tools of Theoretical Physics and Optimal Control to derive unified laws of cognition for learning and inference in recurrent neural networks. I demonstrate that Hamiltonian equations, in their causal dissipative form, lead to a novel neural propagation scheme that is local in both space and time. This addresses the longstanding debate on the biological plausibility of Backpropagation and offers a new framework for developing lifelong learning intelligent agents capable of focusing attention and taking conscious actions.
Interested in Hosting an INNS Webinar?
If you or someone in your professional network are interested in hosting a webinar, please complete the form below.
|